|
ACCESS THE FULL ARTICLE
No SPIE Account? Create one
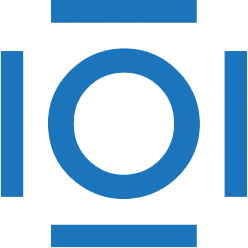
CITATIONS
Cited by 3 scholarly publications.
Image classification
Hyperspectral imaging
Surface plasmons
Image segmentation
Spatial resolution
Associative arrays
Feature extraction