1.IntroductionLaser speckle contrast imaging (LSCI) is an optical imaging technique based on coherent dynamic light scattering that has been widely adopted for visualizing changes in blood flow.1,2 Requiring only a laser for illumination and a camera for detection, LSCI can create full-field maps of motion with high spatiotemporal resolution. This has produced great interest in using LSCI for imaging flow in preclinical neuroscience research3–6 as well as a broad set of clinical applications spanning the skin,7,8 retina,9,10 and brain.11–13 Efforts to improve the quantitative accuracy of LSCI resulted in the development of multi-exposure speckle imaging (MESI),14 which offer more robust estimates of flow compared to traditional single-exposure LSCI.15,16 The acquisition of data at multiple camera exposure times increases the dynamic range of flow sensitivity while the more comprehensive dynamic light scattering model better accounts for the presence of static scatterers and instrumentation factors. These improvements have facilitated the chronic imaging of neurovascular blood flow17–20 and offer increased sensitivity during neurosurgical measurements.21 Due to its popularity, LSCI instrument development is an active area of research with microfluidic studies commonly used to characterize performance in controlled flow environments. Typically, studies designed to mimic blood flow involve the use of a flow channel embedded in a polydimethylsiloxane (PDMS) substrate,14 glass capillary tubing,22 or clear plastic tubing.23 More complex microfluidic networks have also been fabricated in glass, plastic, or epoxy substrates to represent superficial heterogenous vasculature.24 A scattering solution14,22 or whole blood23 is then flowed through the channel at controlled rates and imaged using LSCI. For applications in rodent studies, 0.1 to is a commonly selected range of flow speeds that corresponds with experimentally measured capillary speeds.25 This speed can be converted to a volumetric flow rate using the cross-sectional area of the flow channel, which can then be used to program the flow controller. Syringe pumps are the most widely used flow control system in microfluidic studies and are generally believed to provide a highly controlled flow output. A survey of over 20 LSCI studies using microfluidics that were published since 2015 found that syringe pumps were used in the majority (90%) of experiments. The devices were from a variety of manufacturers and featured various syringe sizes and materials. Syringe pumps operate by applying mechanical force on the plunger of a syringe at a controlled speed. A rotating lead screw driven by a stepper motor controls the velocity of the plunger, which is calculated based on the syringe size and inner diameter to achieve a desired flow rate. However, syringe pumps produce oscillations in flow with varying frequency and magnitude due to the physical motion of the motor and from imperfections on the lead screw.26,27 Syringe pumps have also been shown to have slow responsivity and can take a long time to achieve programmed flow rates.28 The trade-off between stability and responsivity makes it difficult29 to obtain both stable flow and a fast flow response with a syringe pump system, both of which are necessary for LSCI characterization measurements. Pressure-driven flow control systems overcome the limitations of syringe pumps by eliminating the use of a motor and lead screw to provide very stable flow.26,28,30,31 These systems use pressure-regulated air to push liquid from a reservoir through the microfluidic device at a constant flow rate.31 To control the applied pressure, an absolute flow sensor must be added inline with the microfluidic device. Thermal mass flow sensors are commonly used to measure the low flow rates found in microfluidics. These sensors use heating and temperature-sensing elements to detect the magnitude and direction of flow and are factory-calibrated to measure absolute flow rates for various fluids. Integration of the flow sensor with a feedback loop allows for the programming of specific flow rates rather than pressure levels. In this paper, we address two primary objectives. First, we quantify the uncertainty in flow generation for both a syringe pump and pressure-regulated flow system in microfluidic flow phantoms commonly used with optical blood flow imaging instruments. By understanding the real-world uncertainty associated with each flow controller, we can design a microfluidic study to characterize the performance of optical imaging techniques that minimizes the impact of flow-related errors. Second, we use the pressure-regulated flow system to assess the performance of both LSCI and MESI across a range of physiologically-relevant flow speeds. Using the inline flow sensor as reference, we demonstrate the superior accuracy of relative flow measurements with MESI compared to traditional single-exposure LSCI. Such measurements would have been confounded by the large uncertainties associated with syringe pump flow generation. The results of this study indicate that highly-accurate flow controllers, such as pressure-regulated flow systems, should be used when characterizing laser speckle techniques with microfluidic studies. 2.Methods2.1.Microfluidic Phantom FabricationThe microfluidic flow phantom was cast using PDMS (Sylgard 184™, Dow Corning) with 1.8-mg titanium dioxide () added per gram of PDMS to generate a scattering background that mimics tissue optical properties at near infrared wavelengths ().14,32 The PDMS- mixture was vigorously mixed for 15 min to ensure even distribution of the in the phantom. Centrifugation (3000-g relative centrifugal force, 10 min) was then performed to de-gas the sample and remove any clumps. A machined aluminum mold was used to cast the microfluidic as shown in Fig. 1(a). The extrusion is , which formed three walls of the channel. The square cross-section (1:1 aspect ratio) approximates the circular cross-section of vascular geometries in vivo. The PDMS- mixture was carefully poured on top of the mold within a polystyrene petri dish and placed in a vacuum chamber to remove air bubbles introduced during pouring. The PDMS was then cured at 70°C for 1 hour, allowed to cool, and detached from the mold. The microchannel side of the phantom was then oxygen plasma bonded to a glass slide to seal the channel. Inlet and outlet ports were attached using 23 gauge blunt tip needles fitted partially inside the phantom, which were epoxied in place for an air-tight seal. Tygon® microbore tubing (0.02″ inner diameter, Cole-Parmer Instrument Company, LLC) was connected to the blunt tip ports as inlet and outlet tubing. The inlet tubing was connected to the flow control system using fluidic fittings with FEP tubing (IDEX Health and Science, LLC). The outlet tubing drained into a vial, with the tubing end fully submerged in liquid (see S1 in the Supplementary Material). A photograph of the phantom is shown in Fig. 1(b), where the channel is visible inside the -doped PDMS with the glass slide sealing the surface. Fig. 1(a) Photograph of the machined aluminum mold with a extrusion used to cast the PDMS during fabrication of the microfluidic flow phantom. (b) Photograph of the finished microfluidic flow phantom, showing PDMS bonded to a glass slide, with inlet and outlet ports secured using epoxy. Tubing was secured to the ports and connected with the rest of the system using fluidic fittings. ![]() A colloidal mixture of suspended diameter polystyrene microspheres (10% w/w, 5100A, Thermo Fisher Scientific, Inc.) in ultrafiltered deionized (UFDI) water was used as a blood-mimicking solution. Based on Ref. 33, the reduced scattering coefficient () of whole blood with hematocrit of 0.5 is approximately . Using a 7% by volume mixture of the microsphere solution and UFDI water (e.g., 10 mL of blood mimicking solution = 0.7 mL of the 5100A solution + 9.3-mL UFDI water), we were able to achieve a calculated of . However, we note that the anisotropy of the microsphere solution is different than that of whole blood ( versus ). The blood-mimicking solution was preferred for several reasons: cleaning and safety, ease of acquisition, and because the flow sensor was factory-calibrated for deionized water. Because the concentration of microspheres was very low (0.7% by weight in the final suspension), the water calibration of the flow sensor remained valid. The solution of microspheres was freshly mixed before each experiment to reduce particle aggregation. Once mixed, the solution was sonicated for 10 min to fully suspend the particles and then degassed under vacuum to eliminate air bubbles. 2.2.Microfluidic Flow System InstrumentationTo assess the performance of the syringe pump and pressure-regulated flow systems, and to quantify the uncertainty of each system, it is necessary to know the true flow rate of the blood-mimicking solution through the microfluidic channel. A thermal mass flow sensor (FLU-L, , Fluigent, Inc.) was placed inline between the flow control system and the phantom inlet tubing, as shown in Fig. 2(a). This flow rate range corresponds to flow speeds of in the microfluidic channel. The sensor output was relayed to a computer via an accompanying hub (Flowboard, FLB, Fluigent, Inc.) where the MAESFLO software (Fluigent, Inc.) was used to monitor and record the absolute flow rate. All flow sensor measurements were downsampled from 10 to 2 Hz using interpolation to match the timing of the optical measurements. Fig. 2(a) LSCI system schematic for microfluidic flow assessment (L = lens, F = filter). A commercial flow sensor was placed in series with the inlet tubing for the microfluidic flow phantom to obtain real-time absolute flow measurements. Flow was programmatically controlled using either a (b) syringe pump or (c) pressure-regulated flow system. The software for the pressure flow system used a feedback loop between the flow sensor readings and the pressure controller to regulate air pressure. ![]() The syringe pump system (74905-04, Cole-Parmer Instrument Company, LLC) was connected to the flow sensor [Fig. 2(b)] and a 10-mL plastic syringe (#302149, BD) was filled with the blood-mimicking solution described in Sec. 2.1. The syringe pump was programmed to run a predefined flow profile, using the 14.5-mm inner diameter of the syringe to ensure a correct flow rate. It should be noted that a 3-mL syringe was also evaluated, however its performance was worse than the 10-mL syringe and could not hold sufficient volume for all trials without being refilled. The pressure-regulated flow system used a pressure controller (MFCS-EZ with 69 mbar channel, Fluigent, Inc.) connected to a house air line regulated at 500 mbar, as shown in Fig. 2(c). This controller outputted regulated air flow (up to 69 mbar) to a 15-mL pressurized reservoir (Fluiwell-1C, Fluigent, Inc.) filled with the blood-mimicking solution. Prior to running a flow program, a calibration was performed that accounted for the resistance from the tubing, microfluidic channel, and hydrostatic pressure. The MAESFLO control software used feedback from the inline flow sensor to regulate the air pressure to achieve the desired flow rate. 2.3.Microfluidic Flow System AssessmentTwo separate stepped flow profile experiments were conducted to assess each flow system using the commercial flow sensor. First, a three-step flow profile was used to determine the uncertainty of each flow control system. The experiment lasted 240 s, beginning with 30 s of no flow () followed by one full minute at each flow speed (2.4, 3.6, and ) before concluding with an additional 30 s of no flow. The experiment was repeated three times on each system. The second flow profile experiment consisted of a 13-step flow profile. The flow speed was once again varied from 2.4 to , however in smaller increments. As with the first experiment, the flow profile begins and ends with 30 s of no flow, with one full minute at each programmed flow speed (840 s total duration). The second experiment was also repeated three times on each flow control system. To quantify and compare each flow system, we calculated the total combined uncertainty in accordance with National Institute of Standards and Technology guidelines.34,35 Three main sources of uncertainty were identified for inclusion in the uncertainty budget: repeatability, reproducibility, and measurement bias. Standard uncertainties were calculated for each flow step using 55 s of data, allowing for 5 s of transition from the previous flow state. All values were expressed as percentages relative to their respective means. The uncertainty in repeatability, which is a measure of within-run variance, was defined as where is the pooled variance across the three trials at each flow speed and is the mean value of the measured flow speed across all trials.The uncertainty in reproducibility, which is a measure of between-run variance, was defined as where is the variance in the mean flow speed across all three trials and is the mean value of the measured flow speed across all trials.Finally, the uncertainty in measurement bias, an estimate of flow accuracy, was defined as where is the mean value of the measured flow speed across all trials and is the programmed flow speed (2.4, 3.6, or ) at each step.The total combined standard uncertainty () was then calculated using the root sum of squares of the individual uncertainty components and reported as the expanded uncertainty () with a coverage factor corresponding to a level of confidence of . 2.4.LSCI InstrumentationA schematic of the LSCI setup is shown in Fig. 2(a). Measurements were performed using a 660-nm laser diode (HL6545MG, Thorlabs Inc.) mounted in a temperature-controlled housing (LDM21, Thorlabs Inc.) and expanded using an aspheric lens to obliquely illuminate the microfluidic. The laser diode controller (LDC205C, Thorlabs, Inc.) was set to 200 mA () while the temperature controller (TED200C, Thorlabs Inc.) was set to 10.5 kΩ (23.8°C). A pair of fixed focal length consumer camera lenses (AF Nikkor 50-mm , Nikon Corp.) were placed in tandem with infinity focus for 1:1 imaging. The aperture of the lower lens was set to to properly sample the speckle pattern36 while the upper lens was left at its maximum size of . A longpass red filter (600 nm, R-60, Edmund Optics, Inc.) was placed between the two lenses to filter stray background light. The scattered light was imaged with a camera (piA640-210gm, , Basler AG) controlled using custom software. The full sensor array was used, resulting in a field-of-view of . The camera exposure time was set to 0.5 ms and allowed to run at full speed, resulting in an effective acquisition rate of frames-per-second (fps). 2.5.LSCI Flow MeasurementsTo validate the flow sensor measurements, single-exposure LSCI was used to simultaneously image the microfluidic flow phantom during the 13-step flow experiments described in Sec. 2.3. Raw intensity images were converted to speckle contrast images using Eq. (4), where speckle contrast () is defined as the ratio of the standard deviation () to the mean intensity () in a sliding window centered at every pixel of the raw image During post-processing, the average speckle contrast was extracted from each frame within a region centered in the microfluidic channel. This value was used to estimate the correlation time () of the speckle autocorrelation function, which is considered a more quantitative measure of flow.37 The averaged speckle contrast value at each timepoint was fitted for its corresponding using the following equation where is the camera exposure time, , and is an instrumentation factor that accounts for speckle sampling, polarization, and coherence effects.38 For these measurements, it was assumed that , which exclusively allows for the computation of relative flow changes. The resulting timecourse of values was resampled to 2 Hz using interpolation and smoothed temporally with a central moving average filter ().Because is inversely related to the speed of moving scatterers in the sample,39 the inverse correlation time () is commonly used for visualization. To compare the LSCI measurements with the flow sensor, the relative flow for each technique was calculated using the average value of the first step () as the baseline. Because the flow sensor software used a separate clock than the LSCI acquisition, the datasets were synchronized during post-processing using the initial rise of the first flow step. 2.6.MESI InstrumentationA schematic of the MESI setup is shown in Fig. 3. MESI was performed using a wavelength-stabilized 785-nm laser diode (LD785-SEV300, Thorlabs, Inc.) mounted in a temperature-controlled housing (TCLDM9, Thorlabs, Inc.) and collimated using an aspheric lens (C280TMD-B, Thorlabs, Inc.). The operating current on the laser diode controller (LDC205C, Thorlabs, Inc.) was fixed at 380 mA (300 mW) and the target temperature set to 25°C on the temperature controller (TED200C, Thorlabs, Inc.). The collimated laser light was passed through a free-space optical isolator (Electro-Optics Technology, Inc.) to minimize back reflections that interfere with single frequency performance. Because the external volume holographic grating of the stabilized laser diode produces a dark spot in the far field, the laser was coupled into a single-mode fiber optic patch cable (P3-780A-FC-2, Thorlabs, Inc.) to obtain a Gaussian beam. The fiber output was recollimated (F230APC-780, Thorlabs, Inc.), intensity modulated with an acousto-optic modulator (AOM, 3100-125; RF Driver 1110AF-AIFO-1.0, Gooch & Housego, Ltd.), and relayed to obliquely illuminate the microfluidic. Fig. 3System schematic for microfluidic flow assessment of MESI and LSCI measurements using only the pressure-regulated flow system (A = aperture, M = mirror, L = lens, F = filter). ![]() A pair of consumer camera lenses were used to image the scattered light (AF Nikkor 50 mm + AF Micro-Nikkor 105 mm , Nikon Corp.) with magnification through a bandpass filter (, 87-773, Edmund Optics Inc.) to a camera (acA1920-155um, , Basler AG). The aperture of the lower lens was set to while the upper lens was left at its maximum size of . Only a subset of the overall sensor array was used (), resulting in a field-of-view of . The camera exposures were temporally synchronized with the modulated laser pulses from the AOM. Fifteen different camera exposures ranging between and 80 ms were recorded for each complete MESI frame, resulting in an effective acquisition rate of . The total amount of light used to capture each exposure was held constant with the AOM to minimize the effects of shot noise.14 The entire acquisition was controlled using custom software written in C++ integrated with a multifunction I/O device (USB-6363, National Instruments Corp.) for the generation of camera exposure trigger signals and AOM modulation voltages. 2.7.MESI and LSCI PerformanceA stepped flow profile experiment was conducted using the pressure-regulated flow system to assess the performance of both MESI and LSCI using the same optical hardware. The flow profile consisted of 19 steps spanning 1 to in increments. Each speed was maintained for two min with a total experiment duration of 40 min, including brief periods of no flow () at the beginning and end of the profile. MESI measurements were acquired continuously throughout the experiment at the maximum acquisition rate of the system (). Single-exposure (0.5 ms) LSCI frames were extracted from the MESI dataset post hoc. The initial rise of the first flow step was again used to synchronize the timing of the MESI/LSCI and flow sensor datasets. The experiment was repeated a total of four times across 2 days. The raw intensity images were converted to speckle contrast images and the average value within the microfluidic channel extracted from each frame as described previously in Sec. 2.5. The average speckle contrast values from all fifteen exposure times were then fitted to the multi-exposure speckle visibility equation 14 to obtain an estimate of where is the camera exposure time, ; is the fraction of light that is dynamically scattered; is a normalization factor that accounts for speckle sampling; and represents exposure-independent instrument noise and nonergodic variances. was held constant at all timepoints during an experiment by performing an initial fit to Eq. (6) using the median speckle contrast value at each exposure across the entire multi-exposure dataset. All fitting was performed with the Levenberg-Marquardt nonlinear least squares algorithm40 using a custom program written in MATLAB (R2021a, MathWorks, Inc.). The resulting timecourse of values was resampled to 2 Hz using interpolation, smoothed temporally with a central moving average filter (), and inverted to obtain ICT.To directly compare the MESI and single-exposure LSCI measurements with the flow sensor, the relative flow was calculated using the average value of the first step () as the baseline. The average values for each flow step were calculated using 115 s of data, allowing for 5 s of transition from the previous step. 3.Results3.1.Microfluidic Flow System AssessmentThe comparison between the syringe pump and the pressure-regulated flow systems as measured with the inline flow sensor is shown in Fig. 4. These plots demonstrate that the pressure-regulated flow system outperforms the syringe pump by accurately and steadily matching the programmed flow speeds across both experiments. While both flow systems responded quickly to the desired flow changes during the large step size experiment [Fig. 4(a)], the syringe pump system suffered from accuracy and stability issues compared to the pressure-regulated flow system. Furthermore, the small step size experiment [Fig. 4(b)] demonstrated that the syringe pump system could not reliably reproduce the finer flow changes as evidenced by the loss of the stair-stepped flow pattern. Fig. 4Flow sensor measurements for the syringe pump (blue) and pressure-regulated (red) flow systems compared to the ideal programmed flow (black) for one run of the (a) 3-step and (b) 13-step experiments. The microfluidic flow speed varied between 2.4 and in 1.2 and steps for the 3- and 13-step experiments, respectively. ![]() Table 1 shows a comparison of the standard uncertainties for repeatability (), reproducibility (), and bias () for each system at the flow speeds tested in the three-step experiments. These results quantify the observations from Fig. 4 that the pressure-regulated flow system is more stable, repeatable, and accurate at all the tested flow speeds. The combined and expanded uncertainties for both systems are presented in Table 2. These results provide evidence of the superiority of the pressure-regulated flow system, particularly when attempting to produce small flow changes. With the syringe pump system, it would be impossible to reliably separate 20% changes in relative flow from flow system noise at the flow speed. This magnitude of uncertainty in flow generation would make it difficult to assess an optical system’s ability to detect small changes in flow. This limitation is visually represented in Fig. 4(b), where the syringe pump fails to resolve the finer flow changes. Using the pressure-regulated flow system, experiments could be designed with relative flow changes of only 1.25% while remaining confident that the resulting optical system measurements were not caused by flow system noise. This level of certainty would also permit assessing improvements to optical system performance at this scale. Table 1Standard uncertainties for repeatability, reproducibility, and bias by flow speeds for the syringe pump and pressure-regulated flow systems.
Table 2Combined and expanded uncertainties (with k=2 coverage) by flow speeds for the syringe pump and pressure-regulated flow systems.
3.2.LSCI Flow MeasurementsThe comparison between the syringe pump and the pressure-regulated flow systems as measured with the inline flow sensor and single-exposure LSCI using the system described in Sec. 2.4 is shown in Fig. 5. The underlying data for both plots are the same as in Fig. 4(b) but are normalized against the first flow step to permit comparison. The relative flow changes measured by LSCI closely track those of the flow sensor and corroborate the accuracy and stability issues seen with the syringe pump system. These results demonstrate that LSCI is capable of detecting similar changes as the commercial flow sensor when measuring relative flow changes up to double the baseline. 3.3.MESI and LSCI PerformanceThe flow measurement performance assessment using the pressure-regulated flow system is shown in Fig. 6. The relative flow measured by MESI and LSCI using the system described in Sec. 2.6 are plotted alongside the flow sensor measurements from a single trial in Fig. 6(a). All relative flows were calculated using the average of the first flow step () as the baseline. Data for each flow step were pooled and averaged across all four trials to assess the overall performance as seen in Fig. 6(b). The coefficients of determination () for this averaged MESI and LSCI data relative to the flow sensor were 0.988 and 0.839, respectively. The concordance correlation coefficients (), a metric designed to evaluate reproducibility,41 were 0.994 and 0.908, respectively. These results demonstrate both strong agreement between MESI and the flow sensor measurements and that MESI outperforms LSCI across large changes in relative flow. It should be noted that the apparent disparity in LSCI performance between Figs. 5(b) and 6 is primarily the result of different flow steps being used as the baseline in each set of experiments. While not an exact comparison, the LSCI measured flow at the step is the flow at the step in Fig. 6, approximately mirroring the change seen between the 2.4 and steps in Fig. 5(b). Fig. 6(a) Relative flow profiles for the flow sensor (black), LSCI (blue), and MESI (red) for one trial, all normalized to the average of the first flow step (). (b) Performance of LSCI and MESI flow estimates compared to measured microfluidic flow speed aggregated across all four trials (mean ± sd). The dashed line denotes the line of equality indicative of a linear 1:1 relationship. ![]() Figure 7 summarizes the accuracy of the MESI and LSCI measurements at each flow step aggregated across all four trials. The absolute error for MESI was below 5% except for flow speeds between 3.5 and , where it increased to almost 9%. In contrast, the LSCI error was over 8.2% for all flow speeds, increasing to over 17% for flow speeds . Aggregated across all tested flow speeds, the average absolute error for MESI was and LSCI was . 4.DiscussionAlmost all microfluidic studies used for characterizing LSCI and MESI systems have been performed using syringe pumps. This study corroborates previous work26,28,31 that has shown syringe pumps do not produce stable flow outputs and demonstrates that single-exposure LSCI is sensitive enough to detect the resulting flow anomalies (Fig. 5). Furthermore, the close agreement between LSCI and the flow sensor demonstrates that these anomalies could be incorrectly interpreted as noise in the optical imaging system when they are actual flow variations. Such a conclusion would underestimate the true performance of an LSCI or MESI system being evaluated for real-world applications. Compared to the syringe pump, the pressure-regulated flow system produced much more stable and reproducible flow outputs across all trials. Because microfluidic studies are commonly used to assess LSCI and MESI instrument performance, an unstable flow control system contributes additional noise to the measurements, making it more difficult to properly characterize a system. For example, the flow profiles in Fig. 4 and the uncertainty analysis in Table 2 show large errors and high variability within and across trials using the syringe pump. These instabilities in flow were caused entirely by the syringe pump, as independently validated using single-exposure LSCI in Fig. 5(a). Such instability would increase the uncertainty in the true performance of any instrument being evaluated. The same analysis shows that a more reliable flow controller, such as the pressure-regulated flow system, produces minimal error and much improved reproducibility, therefore facilitating a more robust instrument assessment. The measured noise levels quantified by follow the specifications published by the manufacturers of both flow control systems. This indicates that the stability results shown are typical and representative of actual performance differences. The for the syringe pump across all flow steps (up to 1.86%) was within the manufacturer’s tolerances, which state that instantaneous flow should be from the mean value. The for the pressure-regulated flow system (up to 0.55%) slightly exceeded the manufacturer’s specifications, which indicated that the best stability achievable with this system configuration was from the mean value. Much larger differences arose from issues with reproducibility and accuracy, with the and of the syringe pump measuring several orders of magnitude higher than those of the pressure-regulated flow system. The syringe pump greatly exceeded the manufacturer’s tolerances for both reproducibility () and accuracy (). Corresponding specifications were not available for the pressure-regulated flow system. While it is possible that using a glass syringe28 or sending the device for manufacturer recalibration could improve performance on the syringe pump, it is unlikely to ever achieve the stability and reliability seen with the pressure-regulated flow system. The evaluation of MESI and single-exposure LSCI using the pressure-regulated flow system further demonstrates the superiority of the MESI technique.16 The MESI estimates of relative flow outperformed LSCI at all flow speeds and closely followed the flow sensor measurements. This included the periods with no flow at the beginning and end of each experiment, where LSCI still measured relative flow of almost the baseline. As seen in Fig. 7, the largest absolute error for MESI was comparable to that of the smallest absolute error for LSCI. While MESI exhibited slightly more variability during individual flow profile steps (Fig. 6), this was likely caused by the fitting process required to calculate . Numerical artifacts introduced by this nonlinear fitting could also be responsible for the increased error seen with MESI at flow speeds between 3.5 and . It is also possible that discrepancies between MESI and the flow sensor could be attributed to a difference in flow between the microfluidic channel and the inlet line where the flow sensor is located. Such a difference could be caused by air leakage in the inlet or outlet port of the phantom, which would reduce the flow measured by the MESI system without impacting the upstream flow measured by the flow sensor. The linearity of the results seen with both MESI and LSCI suggest that a calibration to absolute flow speeds might be feasible. However, any such calibration would be highly dependent upon experimental parameters, including the sample geometry (i.e. channel size), scatterer concentration, illumination configuration, and exposure time selection. Changes to any of these parameters would produce different ICT values, which would affect the correlation with the flow sensor and invalidate the calibration. It also remains to be seen whether calibrations performed in controlled microfluidic environments can reliably be translated to animal or human subjects since in vivo measurements sample a range of different vessels.42,43 However, if microfluidics are necessary for the calibration process, these results demonstrate that a pressure-regulated flow system should be used to produce stable, repeatable, and accurate flow. While the pressure-regulated flow system outperformed the syringe pump during testing, it has two major disadvantages: increased cost and difficulty of use. For the models used in this study, the pressure-regulated flow system was more expensive than the syringe pump. Although there is variability in pricing for both types of systems depending on the vendor and specific features, pressure-regulated flow systems are consistently the more expensive. They are also considerably more difficult to use and maintain than a syringe pump, which simply requires filling a syringe with solution, securing it to the pump, and specifying the desired flow rate. The setup for a syringe pump can be performed quickly with minimal room for error. In contrast, the pressure-regulated flow system requires an extensive setup process and much greater care must be taken to achieve the stated performance of the device. For example, to maximize stability, tubing lengths must be selected to achieve a certain level of resistance in the system such that the desired flow range (0 to ) spans the available range of the pressure controller (0 to 69 mbar). Furthermore, since fluidic fittings are required for connecting all system components, diagnosing instabilities caused by air leakage from an improperly secured fitting can become a difficult endeavor. The system also requires a calibration procedure before any flow program can be executed. While this helps ensure a high level of performance, it is an additional step during an already lengthy setup process. Overall, the pressure-regulated flow system was more tedious and had much greater potential for error. However, to accurately test instrument sensitivity to small flow changes, the pressure-regulated flow system was the only option with the necessary stability and reliability required to produce such flow changes. 5.ConclusionThis paper demonstrates that flow control system selection is critical for reliably generating flow for microfluidic studies. While ubiquitous in the LSCI community, syringe pumps suffer from poor stability and repeatability that limit their ability to properly characterize the flow sensitivity of optical imaging systems. We show that pressure-regulated flow systems should be used instead of syringe pumps when assessing the performance of MESI or LSCI with microfluidics. We determine that the uncertainty in flow generation with a pressure-regulated flow system is considerably less than that of a syringe pump system across a range of physiologically relevant flow speeds. We use the pressure-regulated flow system to further demonstrate the superiority of MESI compared to traditional single-exposure LSCI. Using an inline flow sensor as reference, we show that MESI offers higher accuracy than LSCI across a wide range of flow speeds, with average errors of 4.6% and 15.7%, respectively. Given the large uncertainties associated with the syringe pump system, it would have been impossible to conclude that such measurements arose from an actual flow change rather than systematic error. Overall, these results indicate that highly accurate flow control is necessary for the reliable characterization of an MESI or LSCI system. AcknowledgmentsThe authors would like to acknowledge Jason R. Cook for machining the aluminum mold used to cast the microfluidic channel. The authors would also like to thank Sertan Kutal Gökçe for providing the oxygen plasma bonding procedure, which was used to fabricate the microfluidic phantom. This study was supported by the National Institutes of Health (Grant Nos. NS108484, EB011556, and EB007507) and the UT Austin Portugal Program. C.T.S., L.M.R., and P.K.M. constructed the imaging system, conceived the experiments, and acquired the data. C.T.S., A.S., L.M.R., P.K.M., and C.S. analyzed the results. C.T.S, A.S., and L.M.R. wrote the manuscript. A.K.D. supervised the project. All authors contributed to manuscript revisions. Data, Materials, and Code AvailabilityThe code and data that support the findings of this study are available from the corresponding author, A.K.D., upon reasonable request. ReferencesJ. D. Briers,
“Laser Doppler, speckle and related techniques for blood perfusion mapping and imaging,”
Physiol. Meas., 22 R35
–R66 https://doi.org/10.1088/0967-3334/22/4/201 PMEAE3 0967-3334
(2001).
Google Scholar
D. A. Boas and A. K. Dunn,
“Laser speckle contrast imaging in biomedical optics,”
J. Biomed. Opt., 15
(1), 011109 https://doi.org/10.1117/1.3285504 JBOPFO 1083-3668
(2010).
Google Scholar
C. Ayata et al.,
“Laser speckle flowmetry for the study of cerebrovascular physiology in normal and ischemic mouse cortex,”
J. Cereb. Blood Flow Metab., 24 744
–755 https://doi.org/10.1097/01.WCB.0000122745.72175.D5
(2004).
Google Scholar
T. Durduran et al.,
“Spatiotemporal quantification of cerebral blood flow during functional activation in rat somatosensory cortex using laser-speckle flowmetry,”
J. Cereb. Blood Flow Metab., 24 518
–525 https://doi.org/10.1097/00004647-200405000-00005
(2004).
Google Scholar
H. Nakamura et al.,
“Spreading depolarizations cycle around and enlarge focal ischaemic brain lesions,”
Brain, 133 1994
–2006 https://doi.org/10.1093/brain/awq117 BRAIAK 0006-8950
(2010).
Google Scholar
F. He et al.,
“Multimodal mapping of neural activity and cerebral blood flow reveals long-lasting neurovascular dissociations after small-scale strokes,”
Sci. Adv., 6 eaba1933 https://doi.org/10.1126/sciadv.aba1933 STAMCV 1468-6996
(2020).
Google Scholar
M. Roustit et al.,
“Excellent reproducibility of laser speckle contrast imaging to assess skin microvascular reactivity,”
Microvasc. Res., 80
(3), 505
–511 https://doi.org/10.1016/j.mvr.2010.05.012 MIVRA6 0026-2862
(2010).
Google Scholar
G. Mahé et al.,
“Assessment of skin microvascular function and dysfunction with laser speckle contrast imaging,”
Circ. Cardiovasc. Imaging, 5
(1), 155
–163 https://doi.org/10.1161/CIRCIMAGING.111.970418
(2012).
Google Scholar
J. D. Briers and A. F. Fercher,
“Retinal blood-flow visualization by means of laser speckle photography,”
Invest. Ophthalmol. Visual Sci., 22
(2), 255
–259 IOVSDA 0146-0404
(1982).
Google Scholar
X. Feng et al.,
“Functional imaging of human retina using integrated multispectral and laser speckle contrast imaging,”
J. Biophotonics, 15 e202100285 https://doi.org/10.1002/jbio.202100285
(2022).
Google Scholar
A. B. Parthasarathy et al.,
“Laser speckle contrast imaging of cerebral blood flow in humans during neurosurgery: a pilot clinical study,”
J. Biomed. Opt., 15
(6), 066030 https://doi.org/10.1117/1.3526368 JBOPFO 1083-3668
(2010).
Google Scholar
E. Klijn et al.,
“Laser speckle imaging identification of increases in cortical microcirculatory blood flow induced by motor activity during awake craniotomy,”
J. Neurosurg., 118 280
–286 https://doi.org/10.3171/2012.10.JNS1219 JONSAC 0022-3085
(2013).
Google Scholar
D. R. Miller et al.,
“Continuous blood flow visualization with laser speckle contrast imaging during neurovascular surgery,”
Neurophotonics, 9
(2), 021908 https://doi.org/10.1117/1.NPh.9.2.021908
(2022).
Google Scholar
A. B. Parthasarathy et al.,
“Robust flow measurement with multi-exposure speckle imaging,”
Opt. Express, 16 1975
–1989 https://doi.org/10.1364/OE.16.001975 OPEXFF 1094-4087
(2008).
Google Scholar
A. B. Parthasarathy, S. M. S. Kazmi and A. K. Dunn,
“Quantitative imaging of ischemic stroke through thinned skull in mice with Multi Exposure Speckle Imaging,”
Biomed. Opt. Express, 1
(1), 246
–259 https://doi.org/10.1364/BOE.1.000246 BOEICL 2156-7085
(2010).
Google Scholar
S. M. S. Kazmi et al.,
“Chronic imaging of cortical blood flow using Multi-Exposure Speckle Imaging,”
J. Cereb. Blood Flow Metab., 33 798
–808 https://doi.org/10.1038/jcbfm.2013.57
(2013).
Google Scholar
C. J. Schrandt et al.,
“Chronic monitoring of vascular progression after ischemic stroke using multiexposure speckle imaging and two-photon fluorescence microscopy,”
J. Cereb. Blood Flow Metab., 35 933
–942 https://doi.org/10.1038/jcbfm.2015.26
(2015).
Google Scholar
T. A. Clark,
“Artery targeted photothrombosis widens the vascular penumbra, instigates peri-infarct neovascularization and models forelimb impairments,”
Sci. Rep., 9 2323 https://doi.org/10.1038/s41598-019-39092-7 SRCEC3 2045-2322
(2019).
Google Scholar
M. R. Williamson et al.,
“A window of vascular plasticity coupled to behavioral recovery after stroke,”
J. Neurosci., 40 7651
–7667 https://doi.org/10.1523/JNEUROSCI.1464-20.2020 JNRSDS 0270-6474
(2020).
Google Scholar
C. T. Sullender et al.,
“Dynamics of isoflurane-induced vasodilation and blood flow of cerebral vasculature revealed by multi-exposure speckle imaging,”
J. Neurosci. Methods, 366 109434 https://doi.org/10.1016/j.jneumeth.2021.109434 JNMEDT 0165-0270
(2022).
Google Scholar
L. M. Richards et al.,
“Intraoperative multi-exposure speckle imaging of cerebral blood flow,”
J. Cereb. Blood Flow Metab., 37 3097
–3109 https://doi.org/10.1177/0271678X16686987
(2017).
Google Scholar
J. A. Arias-Cruz et al.,
“Visualization of in vitro deep blood vessels using principal component analysis based laser speckle imaging,”
Biomed. Opt. Express, 10
(4), 2020
–2031 https://doi.org/10.1364/BOE.10.002020 BOEICL 2156-7085
(2019).
Google Scholar
P. Miao et al.,
“Entropy analysis reveals a simple linear relation between laser speckle and blood flow,”
Opt. Lett., 39 3907
–3910 https://doi.org/10.1364/OL.39.003907 OPLEDP 0146-9592
(2014).
Google Scholar
L. Luu et al.,
“Microfluidics based phantoms of superficial vascular network,”
Biomed. Opt. Express, 3
(6), 1350
–1364 https://doi.org/10.1364/BOE.3.001350 BOEICL 2156-7085
(2012).
Google Scholar
M. Tomita et al.,
“Automated method for tracking vast numbers of FITC-labeled RBCs in microvessels of rat brain in vivo using a high-speed confocal microscope system,”
Microcirculation, 15 163
–174 https://doi.org/10.1080/10739680701567089 MCCRD8 0275-4177
(2008).
Google Scholar
Z. Li et al.,
“Syringe-pump-induced fluctuation in all-aqueous microfluidic system implications for flow rate accuracy,”
Lab Chip, 14 744
–749 https://doi.org/10.1039/c3lc51176f LCAHAM 1473-0197
(2014).
Google Scholar
W. Zeng et al.,
“Characterization of syringe-pump-driven induced pressure fluctuations in elastic microchannels,”
Lab Chip, 15
(4), 1110
–1115 https://doi.org/10.1039/C4LC01347F LCAHAM 1473-0197
(2015).
Google Scholar
J. Zhou et al.,
“Pumping-induced perturbation of flow in microfluidic channels and its implications for on-chip cell culture,”
Lab Chip, 11 2288
–2294 https://doi.org/10.1039/c0lc00466a LCAHAM 1473-0197
(2011).
Google Scholar
Y. J. Kang and S. Yang,
“Fluidic low pass filter for hydrodynamic flow stabilization in microfluidic environments,”
Lab Chip, 12
(10), 1881
–1889 https://doi.org/10.1039/c2lc21163g LCAHAM 1473-0197
(2012).
Google Scholar
K. W. Bong et al.,
“Compressed-air flow control system,”
Lab Chip, 11 743
–747 https://doi.org/10.1039/C0LC00303D LCAHAM 1473-0197
(2011).
Google Scholar
W. Zeng and H. Fu,
“Precise measurement and control of the pressure-driven flows for microfluidic systems,”
Electrophoresis, 41
(10–11), 852
–859 https://doi.org/10.1002/elps.201900103 ELCTDN 0173-0835
(2020).
Google Scholar
A. N. Yaroslavsky et al.,
“Optical properties of selected native and coagulated human brain tissues in vitro in the visible and near infrared spectral range,”
Phys. Med. Biol., 47 2059
–2073 https://doi.org/10.1088/0031-9155/47/12/305 PHMBA7 0031-9155
(2002).
Google Scholar
A. Roggan et al.,
“Optical properties of circulating human blood in the wavelength range 400-2500 nm,”
J. Biomed. Opt., 4
(1), 36
–47 https://doi.org/10.1117/1.429919 JBOPFO 1083-3668
(1999).
Google Scholar
B. N. Taylor and C. E. Kuyatt, Guidelines for evaluating and expressing the uncertainty of NIST measurement results Gaithersburg, MD
(1994). Google Scholar
C. M. Croarkin,
“Measurement process characterization,”
NIST/SEMATECH e-Handbook of Statistical Methods, NIST Interagency/Internal Report (NISTIR), Gaithersburg, MD
(2002). Google Scholar
S. J. Kirkpatrick, D. D. Duncan and E. M. Wells-Gray,
“Detrimental effects of speckle-pixel size matching in laser speckle contrast imaging,”
Opt. Lett., 33 2886
–2888 https://doi.org/10.1364/OL.33.002886 OPLEDP 0146-9592
(2008).
Google Scholar
J. D. Briers and S. Webster,
“Laser speckle contrast analysis (LASCA): a nonscanning, full-field technique for monitoring capillary blood flow,”
J. Biomed. Opt., 1
(2), 174
–180 https://doi.org/10.1117/12.231359 JBOPFO 1083-3668
(1996).
Google Scholar
R. Bandyopadhyay et al.,
“Speckle-visibility spectroscopy: a tool to study time-varying dynamics,”
Rev. Sci. Instrum., 76 093110 https://doi.org/10.1063/1.2037987 RSINAK 0034-6748
(2005).
Google Scholar
R. Bonner and R. Nossal,
“Model for laser Doppler measurements of blood flow in tissue,”
Appl. Opt., 20
(12), 2097
–2107 https://doi.org/10.1364/AO.20.002097 APOPAI 0003-6935
(1981).
Google Scholar
M. I. A. Lourakis,
“levmar: Levenberg-Marquardt nonlinear least squares algorithms in C/C++,”
(2004). Google Scholar
L. I.-K. Lin,
“A concordance correlation coefficient to evaluate reproducibility,”
Biometrics, 45 255
–268 https://doi.org/10.2307/2532051 BIOMB6 0006-341X
(1989).
Google Scholar
M. A. Davis et al.,
“Sensitivity of laser speckle contrast imaging to flow perturbations in the cortex,”
Biomed. Opt. Express, 7 759
–775 https://doi.org/10.1364/BOE.7.000759 BOEICL 2156-7085
(2016).
Google Scholar
C. Z. Jafari et al.,
“Establishing the effect of vascular structure on laser speckle contrast imaging,”
Biomed. Opt. Express, 11 5826
–5841 https://doi.org/10.1364/BOE.401235 BOEICL 2156-7085
(2020).
Google Scholar
BiographyColin T. Sullender is a postdoctoral fellow at the University of Texas at Austin. His research interest includes developing laser speckle contrast imaging techniques for studying neurovascular hemodynamics. Adam Santorelli received his BEng, MSE, and PhD degrees in electrical engineering from McGill University. Currently, he is a research associate at the University of Texas at Austin. He was an IRC Government of Ireland postdoctoral fellow in the Translational Medical Device Lab at the National University of Ireland, Galway. His research interest includes the translational aspects of speckle imaging. Lisa M. Richards received her BSE degree in biomedical engineering from Duke University and her MSE and PhD degrees in biomedical engineering from the University of Texas at Austin. She was a postdoctoral fellow at the University of Texas at Austin when this research was conducted. Pawan K. Mannava received his BS degree in biomedical engineering from the University of Texas at Austin. He was an undergraduate researcher at the University of Texas at Austin when this research was conducted. Christopher Smith received his BS degree in biomedical engineering from the University of Houston. He is a graduate student at the University of Texas at Austin. Andrew K. Dunn is the Edward S. Hyman Endowed Chair in the Department of Biomedical Engineering and Director of the Center for Emerging Imaging Technologies (CEIT) at the University of Texas at Austin. He pioneered the use of laser speckle contrast imaging to measure blood flow in the brain. His research interest includes the development of novel optical imaging techniques for studying the brain. |
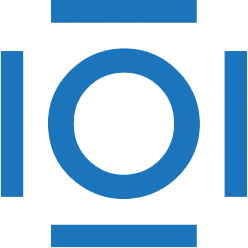
CITATIONS
Cited by 1 scholarly publication.
Laser speckle contrast imaging
Microfluidics
Sensors
Imaging systems
Laser systems engineering
Control systems
Equipment