|
ACCESS THE FULL ARTICLE
No SPIE Account? Create one
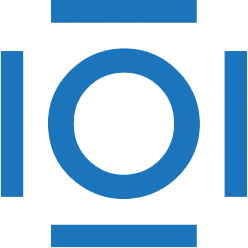
CITATIONS
Cited by 7 scholarly publications.
Video
Data modeling
RGB color model
Remote sensing
Feature extraction
Neurons
Performance modeling