|
ACCESS THE FULL ARTICLE
No SPIE Account? Create one
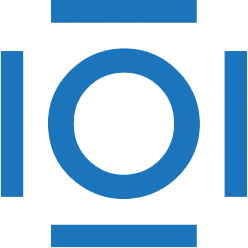
CITATIONS
Cited by 1 scholarly publication.
Image analysis
Neurons
Quantization
Image classification
Data modeling
Convolutional neural networks
Feature extraction