|
ACCESS THE FULL ARTICLE
No SPIE Account? Create one
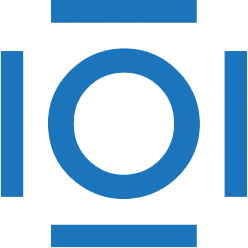
CITATIONS
Cited by 2 scholarly publications.
Video
Lawrencium
Super resolution
Convolution
Optical flow
Convolutional neural networks
Reconstruction algorithms