Monocular visual odometry and depth estimation play an important role in augmented reality and robot applications. Recently, deep learning technologies have been widely used in these areas. However, most existing works utilize supervised learning, which requires large amounts of labeled data and assumes that the scene is static. We propose a framework, called Un-VDNet, based on unsupervised convolutional neural networks to predict camera ego-motion and depth maps from image sequences. The framework includes three subnetworks (PoseNet, DepthNet, and FlowNet) and learns temporal motion and spatial association information in an end-to-end network. We propose a pose-consistency loss to penalize errors about the translation and rotation drifts of the pose estimated from the PoseNet. Furthermore, a geometric consistency loss, between the structure flow and scene flow learned from the FlowNet, is proposed to deal with dynamic objects in the real-world scene, which is combined with spatial and temporal photometric consistency constraints. Extensive experiments on the KITTI and TUM datasets demonstrate that our proposed Un-VDNet outperforms the state-of-the-art methods for visual odometry and depth estimation in dealing with dynamic objects of outdoor and indoor scenes. |
ACCESS THE FULL ARTICLE
No SPIE Account? Create one
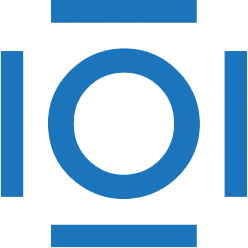
CITATIONS
Cited by 2 scholarly publications.
Visualization
Cameras
Error analysis
Optical flow
Networks
Atomic force microscopy
Convolutional neural networks