|
ACCESS THE FULL ARTICLE
No SPIE Account? Create one
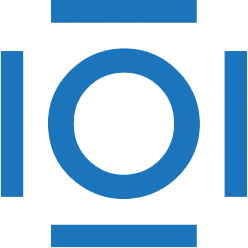
CITATIONS
Cited by 14 scholarly publications.
Particle swarm optimization
Particles
Roentgenium
Illumination engineering
Light sources
Optical engineering
Performance modeling