|
ACCESS THE FULL ARTICLE
No SPIE Account? Create one
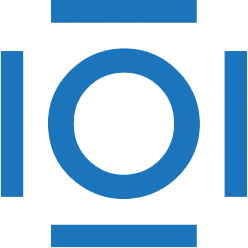
CITATIONS
Cited by 4 scholarly publications.
Lung
Video
Computed tomography
Chest
Computer aided diagnosis and therapy
Lung cancer
3D modeling