Background: As the design layout of integrated circuits (ICs) is continually scaling down, sub-resolution assist features (SRAF) have been extensively used in resolution enhancement technique applications to enhance lithography printing fidelity and widen the manufacturing process window (PW). With conventional SRAF insertion techniques, rule-based SRAF (RB-SRAF) and model-based SRAF (MB-SRAF) methods have since been widely adopted. Aim: The typical RB-SRAF is an efficient method to generate SRAFs consistently for simple designs but cannot be optimized for multiple critical patterns or complex layout schemes. Although MB-SRAF is able to achieve better PW as well as reducing conflicts between placement rules and clean-up rules, many iterations for convergence and extremely high computational costs are required. The explosion of machine learning techniques could facilitate the complex processes of mask optimization, such as SRAF insertion. Approach: Generative adversarial network was studied on a Via layer of advanced 3D NAND flash memory, by training target images and Inverse Lithography Technology (ILT) images of target patterns. GAN models, pix2pix, and CycleGAN, were first trained and then utilized to synthesize realistic ILT images. Results: These ILT images were eventually translated to polygons of SRAF with simplification process and mask manufacturing rules check constraints. The simulation results demonstrate that CycleGAN approach can place SRAF with comparable performance to mask optimization (MO) result which was optimized by the Tachyon source-mask optimizer (SMO). Conclusions: Methodologies of SRAF placement based on GANs were demonstrated in this paper. While traditional MO approach showed the best lithography performance, CycleGAN in particular was close behind and had much better performance than conventional RB-SRAF approach, whilst achieving good stability for different layout environments. Most importantly, the efficiency of SRAF insertion can be enhanced significantly through GANs. |
ACCESS THE FULL ARTICLE
No SPIE Account? Create one
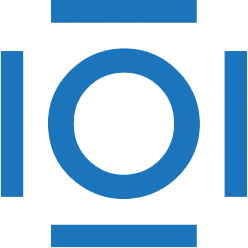
CITATIONS
Cited by 1 scholarly publication.
SRAF
Gallium nitride
Data modeling
Machine learning
Molybdenum
Lithography
Performance modeling